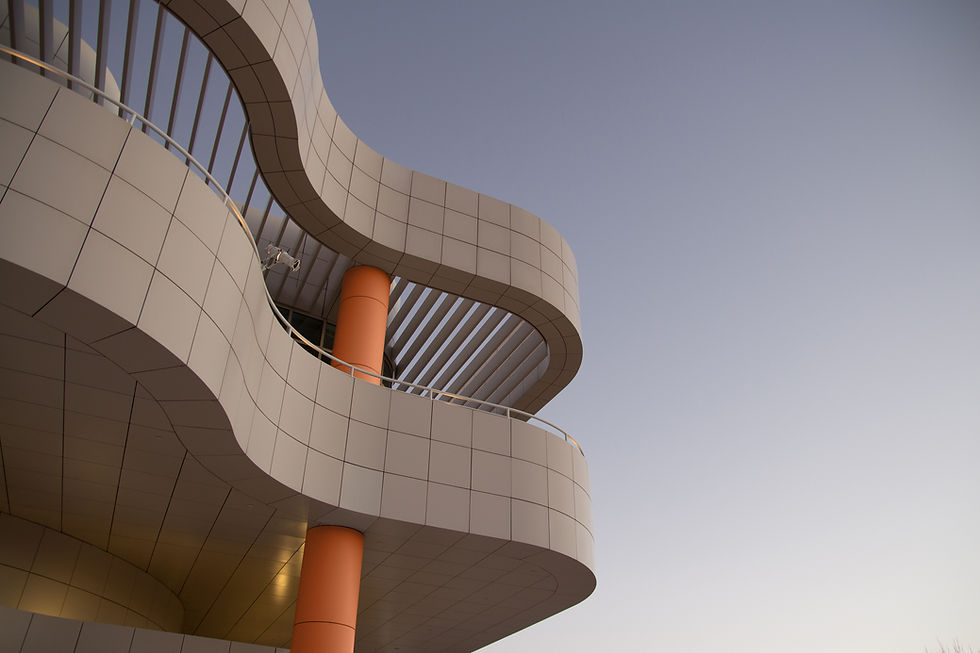
The Hard Truths of AI Adoption: What Works and What Doesn’t
Feb 27
3 min read
0
2
0
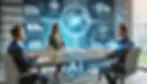
AI Adoption: A Reality Check
As I prepare for my upcoming workshop, AI for Business Leaders, I find myself wondering: Do attendees see AI as a silver bullet for all their business challenges? Will they become frustrated when their proof-of-concept (POC) stalls? Will they remain open-minded and explore alternative ways to leverage AI? Or will they abandon their AI ambitions instead of diagnosing why the technology didn’t meet their expectations?
AI adoption isn’t struggling because of a lack of technology—it’s failing due to misalignment, weak execution, and the ongoing challenge of connecting AI to tangible business value. This edition of AI Unplugged offers a no-nonsense take on what works in AI strategy—and what doesn’t—grounded in real-world lessons.
One standout case is Neeta Upadhyay, Head of Data and Advanced Analytics at Navista, Cardinal Health. Neeta’s role in streamlining a complex network of suppliers, manufacturers, and customers to ensure the right medicine reaches the right place at the right time is an enormous AI enablement challenge. How did Cardinal Health stay on track? What kept their AI adoption from going off the rails? Neeta’s approach to meeting Cardinal ’s vision offers crucial lessons.
The Human-Centric Solution to AI Adoption
🚀 Executive buy-in matters more than technology.
🤝 AI isn’t just an IT project—collaboration is non-negotiable.
📊 Rethinking AI success: It’s not just about models.
1. The Foundation of Strategy—Executive Sponsorship
As AI projects scale, so do expectations. Unlike a decade ago when securing executive buy-in was a hurdle, today’s C-suite is actively exploring AI—especially GenAI—to understand its revenue and profitability implications. But executive enthusiasm alone is not enough.
"Lucky are the organizations where the executive team can descend from their lofty vision and engage in the pragmatic integration of AI."
Neeta’s experience underscores this reality. Having a seat at the table allowed her to engage directly with the C-suite, reset expectations, and drive prioritization around AI challenges. This alignment ensured AI remained a business enabler—not just a technology experiment.
💡 Lesson from the field: If AI teams fail to secure business involvement, projects will struggle to gain traction. Having business sponsors always at the table enabled Neeta to communicate AI’s value effectively and navigate roadblocks with leadership support.
2. The Power of Communication, Collaboration, and Feedback
I often repeat this mantra: Communication, Collaboration, Feedback.
AI today is not just a model deployment exercise—it’s a complex ecosystem of technology, tools, processes, data, and people. The best AI solutions are co-owned by business stakeholders, not just IT. Shared accountability fosters commitment, ensures proper feedback loops, and drives long-term success.
Neeta’s story proves that establishing communication channels early with business peers prevents teams from building solutions “no one asked for or knows how to use.”
📌 On the importance of human oversight: While AI can enhance human decision-making, it should never replace it. A human component must always be connected to whatever we’re optimizing.
A Personal Lesson: The AI Forecasting Challenge
After completing my PhD, my first job was as a global forecasting lead at AT&T. I was eager to apply my time-series models to forecast demand across 240 countries. However, I quickly learned that the accuracy of my models mattered far less than the business impact of my forecasts.
Each month, I had to explain why I missed my forecast. The issue wasn’t the ARIMA model I used—it was the lack of feedback loops with country managers, absence of competitive intelligence, and missing real-world insights that could have explained demand fluctuations.
By collaborating with the finance team, I was able to establish feedback mechanisms, integrate competitive analysis, and provide better insights that leadership could act upon.
💡 Lesson from the field: AI projects don’t fail because of bad models—they fail due to misalignment with business priorities, weak execution, and poor governance.
The Real AI Challenge: Execution, Not Innovation
AI’s biggest challenge isn’t technological innovation—it’s getting organizations to work together to make AI stick.
The focus must shift from “How do we implement AI?” to “How do we integrate AI into business value streams?”
🔎 Tip to Go: If you're struggling with AI execution, start by answering these questions:
Who owns the AI strategy beyond IT?
What business problems does AI actually solve for your company?
Are AI projects being prioritized based on impact—or just hype?
Stay tuned for the next edition of AI Unplugged, where we’ll tackle AI Governance: Ensuring Ethical, Compliant, and Impactful AI Adoption.
📎 Further Reading:
#AIinPharma #PharmaSupplyChain #SmartPharmaceuticals #HealthcareAI #AIExecution #DigitalTransformation #FutureOfAI #AIForBusiness